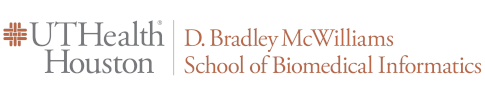
Dissertations & Theses (Open Access)
An Informatics Framework for Representing, Detecting, and Measuring Citations of Biomedical Datasets
Graduation Date
Spring 2024
Degree Name
Doctor of Philosophy (PhD)
School Name
McWilliams School of Biomedical Informatics at UTHealth Houston
Advisory Committee
Kirk Roberts, PhD
Abstract
Biomedical research is inherently data-intensive, emphasizing the necessity of making data findable, accessible, interoperable, and reusable (FAIR) to expedite scientific breakthroughs. Despite the importance, data creators often do not receive sufficient recognition and lack the motivation to publish datasets in accordance with FAIR principles, primarily due to inadequate incentives. Citations serve as a means to attribute credit for dataset creation, track dataset usage, and assess the impact of data. Implementing standardized data citations could significantly enhance the discoverability of data and foster the maintenance of high-quality datasets. Initiatives like DataCite and the FAIRsharing community have been instrumental in addressing this challenge by offering guidelines and resources to support data citation. However, the adherence to these guidelines and proper citation of data in scientific publications remain inconsistent among scientists. This highlights the need for an effective approach to standardize and scrutinize the vast amounts of data citations that fall short of adhering to established guidelines, ensuring they contribute constructively to the scientific community.
One immediate goal is to understand the patterns of current data citation practices and identify high-quality, reliable datasets mentioned in biomedical publications with minimal human efforts. To achieve this, we propose the creation of an informatics framework designed to represent, detect, and evaluate the impact of citations of biomedical datasets effectively. This involves initially developing an information schema to accurately represent biomedical datasets and their attributes as cited in publications.
We then apply this schema to COVID-19 genomic data, annotating citations within COVID-19-related publications to build a corpus that identifies dataset mentions found in biomedical literature. Leveraging this corpus, we advance our methodology by crafting a transformer-based information extraction pipeline. This pipeline is adept at identifying specific mentions of data citation information within the full texts of articles hosted on PubMed Central. Following this, we implement an entity linking strategy that associates mentions of datasets with unique identifiers in biomedical data repositories. The culmination of our efforts is the construction of a comprehensive data-paper citation network. This network integrates all dataset and paper citations, supported by a visualization interface that facilitates the exploration of the network. Through this interface, we conduct an analysis to gauge the impact of datasets, considering both direct and indirect citation relationships within the network, thus providing an in-depth understanding of their significance in the scientific community.
Following the implementation of our informatics framework, we observed significant outcomes. Our NLP pipeline uncovers 11,901 dataset citations across 7,056 COVID-19-related publications. The constructed data-paper citation network comprises 10,908 unique datasets and 289,056 papers, revealing significant trends in data usage and citation patterns. By facilitating easier access to high-quality reusable datasets, researchers can easily leverage existing data for new scientific breakthroughs, fostering a better environment of collaboration and innovation.
Recommended Citation
Zuo, Xu, "An Informatics Framework for Representing, Detecting, and Measuring Citations of Biomedical Datasets" (2024). Dissertations & Theses (Open Access). 61.
https://digitalcommons.library.tmc.edu/uthshis_dissertations/61
Keywords
Data citations, COVID-19 genomic data, extraction pipeline, Natural language processing, interoperability
Comments
This dissertation is also published in the following article: