Publication Date
4-1-2024
Journal
Journal of Biomedical Optics
DOI
10.1117/1.JBO.29.4.046001
PMID
38585417
PMCID
PMC10993061
PubMedCentral® Posted Date
4-4-2024
PubMedCentral® Full Text Version
Post-Print
Published Open-Access
yes
Keywords
Humans, Deep Learning, Optical Fibers, Esophageal Neoplasms, Barrett Esophagus, Image Processing, Computer-Assisted, end-expandable optical fiber probe, deep-learning-based super-resolution, degradation model, Barrett’s esophagus, esophageal cancer, microendoscopy, endomicroscopy
Abstract
SIGNIFICANCE: Endoscopic screening for esophageal cancer (EC) may enable early cancer diagnosis and treatment. While optical microendoscopic technology has shown promise in improving specificity, the limited field of view (
AIM: To improve the efficiency of endoscopic screening, we propose a novel concept of end-expandable endoscopic optical fiber probe for larger field of visualization and for the first time evaluate a deep-learning-based image super-resolution (DL-SR) method to overcome the issue of limited sampling capability.
APPROACH: To demonstrate feasibility of the end-expandable optical fiber probe, DL-SR was applied on simulated low-resolution microendoscopic images to generate super-resolved (SR) ones. Varying the degradation model of image data acquisition, we identified the optimal parameters for optical fiber probe prototyping. The proposed screening method was validated with a human pathology reading study.
RESULTS: For various degradation parameters considered, the DL-SR method demonstrated different levels of improvement of traditional measures of image quality. The endoscopists' interpretations of the SR images were comparable to those performed on the high-resolution ones.
CONCLUSIONS: This work suggests avenues for development of DL-SR-enabled sparse image reconstruction to improve high-yield EC screening and similar clinical applications.
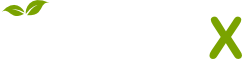
- Citations
- Citation Indexes: 1
- Usage
- Downloads: 3
- Abstract Views: 1
- Captures
- Readers: 34
- Mentions
- News Mentions: 1
Included in
Biomedical Informatics Commons, Digestive System Diseases Commons, Gastroenterology Commons, Medical Sciences Commons, Oncology Commons