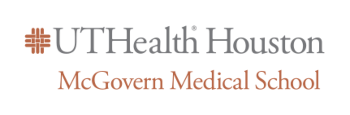
Faculty, Staff and Student Publications
Improved Cerebellar Tissue Classification On Magnetic Resonance Images of Brain
Publication Date
5-1-2009
Journal
Journal of Magnetic Resonance Imaging
Abstract
PURPOSE: To develop and implement a method for improved cerebellar tissue classification on the MRI of brain by automatically isolating the cerebellum prior to segmentation.
MATERIALS AND METHODS: Dual fast spin echo (FSE) and fluid attenuation inversion recovery (FLAIR) images were acquired on 18 normal volunteers on a 3 T Philips scanner. The cerebellum was isolated from the rest of the brain using a symmetric inverse consistent nonlinear registration of individual brain with the parcellated template. The cerebellum was then separated by masking the anatomical image with individual FLAIR images. Tissues in both the cerebellum and rest of the brain were separately classified using hidden Markov random field (HMRF), a parametric method, and then combined to obtain tissue classification of the whole brain. The proposed method for tissue classification on real MR brain images was evaluated subjectively by two experts. The segmentation results on Brainweb images with varying noise and intensity nonuniformity levels were quantitatively compared with the ground truth by computing the Dice similarity indices.
RESULTS: The proposed method significantly improved the cerebellar tissue classification on all normal volunteers included in this study without compromising the classification in remaining part of the brain. The average similarity indices for gray matter (GM) and white matter (WM) in the cerebellum are 89.81 (+/-2.34) and 93.04 (+/-2.41), demonstrating excellent performance of the proposed methodology.
CONCLUSION: The proposed method significantly improved tissue classification in the cerebellum. The GM was overestimated when segmentation was performed on the whole brain as a single object.
Keywords
Adult, Algorithms, Artificial Intelligence, Cerebellum, Female, Humans, Image Enhancement, Image Interpretation, Computer-Assisted, Imaging, Three-Dimensional, Male, Middle Aged, Pattern Recognition, Automated, Reproducibility of Results, Sensitivity and Specificity
DOI
10.1002/jmri.21734
PMID
19388122
PMCID
PMC2678241
PubMedCentral® Posted Date
May 2009
PubMedCentral® Full Text Version
Author MSS
Published Open-Access
yes