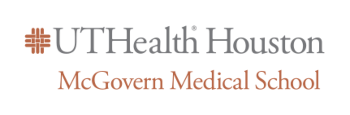
Faculty, Staff and Student Publications
Publication Date
7-21-2023
Journal
BMC Medical Informatics and Decision Making
Abstract
BACKGROUND: Monitoring blood pressure and peripheral capillary oxygen saturation plays a crucial role in healthcare management for patients with chronic diseases, especially hypertension and vascular disease. However, current blood pressure measurement methods have intrinsic limitations; for instance, arterial blood pressure is measured by inserting a catheter in the artery causing discomfort and infection.
METHOD: Photoplethysmogram (PPG) signals can be collected via non-invasive devices, and therefore have stimulated researchers' interest in exploring blood pressure estimation using machine learning and PPG signals as a non-invasive alternative. In this paper, we propose a Transformer-based deep learning architecture that utilizes PPG signals to conduct a personalized estimation of arterial systolic blood pressure, arterial diastolic blood pressure, and oxygen saturation.
RESULTS: The proposed method was evaluated with a subset of 1,732 subjects from the publicly available ICU dataset MIMIC III. The mean absolute error is 2.52 ± 2.43 mmHg for systolic blood pressure, 1.37 ± 1.89 mmHg for diastolic blood pressure, and 0.58 ± 0.79% for oxygen saturation, which satisfies the requirements of the Association of Advancement of Medical Instrumentation standard and achieve grades A for the British Hypertension Society standard.
CONCLUSIONS: The results indicate that our model meets clinical standards and could potentially boost the accuracy of blood pressure and oxygen saturation measurement to deliver high-quality healthcare.
Keywords
Humans, Arterial Pressure, Blood Pressure, Deep Learning, Photoplethysmography, Arteries, Hypertension
DOI
10.1186/s12911-023-02215-2
PMID
37480040
PMCID
PMC10362790
PubMedCentral® Posted Date
July 2023
PubMedCentral® Full Text Version
Post-print
Published Open-Access
yes
Comments
Associated Data
PMID: 37480040