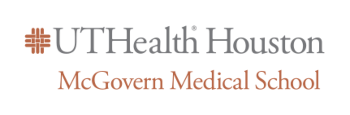
Faculty, Staff and Student Publications
Publication Date
6-1-2023
Journal
Medical Care
Abstract
BACKGROUND: Older adults frequently return to the emergency department (ED) within 30 days of a visit. High-risk patients can differentially benefit from transitional care interventions. Latent class analysis (LCA) is a model-based method used to segment the population and test intervention effects by subgroup.
OBJECTIVES: We aimed to identify latent classes within an older adult population from a randomized controlled trial evaluating the effectiveness of an ED-to-home transitional care program and test whether class membership modified the intervention effect.
RESEARCH DESIGN: Participants were randomized to receive the Care Transitions Intervention or usual care. Study staff collected outcomes data through medical record reviews and surveys. We performed LCA and logistic regression to evaluate the differential effects of the intervention by class membership.
SUBJECTS: Participants were ED patients (age 60 y and above) discharged to a community residence.
MEASURES: Indicator variables for the LCA included clinically available and patient-reported data from the initial ED visit. Our primary outcome was ED revisits within 30 days. Secondary outcomes included ED revisits within 14 days, outpatient follow-up within 7 and 30 days, and self-management behaviors.
RESULTS: We interpreted 6 latent classes in this study population. Classes 1, 4, 5, and 6 showed a reduction in ED revisit rates with the intervention; classes 2 and 3 showed an increase in ED revisit rates. In class 5, we found evidence that the intervention increased outpatient follow-up within 7 and 30 days (odds ratio: 1.81, 95% CI: 1.13-2.91; odds ratio: 2.24, 95% CI: 1.25-4.03).
CONCLUSIONS: Class membership modified the intervention effect. Population segmentation is an important step in evaluating a transitional care intervention.
Keywords
latent class analysis, care transitions, emergency department, healthcare utilization
Comments
PMID: 37167559