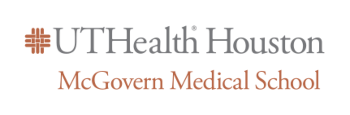
Faculty, Staff and Student Publications
Publication Date
3-1-2023
Journal
Journal of Affective Disorders
Abstract
BACKGROUND: Artificial intelligence is currently being used to facilitate early disease detection, better understand disease progression, optimize medication/treatment dosages, and uncover promising novel treatments and potential outcomes.
METHODS: Utilizing the Sequenced Treatment Alternatives to Relieve Depression (STAR*D) dataset, we built a machine learning model to predict depression remission rates using same clinical data as features for each of the first three antidepressant treatment steps in STAR*D. We only used early treatment data (baseline and first follow up) in each STAR*D step to temporally analyze predictive features of remission at the end of the step.
RESULTS: Our model showed significant prediction performance across the three treatment steps, At step 1, Model accuracy was 66 %; sensitivity-65 %, specificity-67 %, positive predictive value (PPV)-65.5 %, and negative predictive value (NPV)-66.6 %. At step 2, model accuracy was 71.3 %, sensitivity-74.3 %, specificity-69 %, PPV-64.5 %, and NPV-77.9 %. At step 3, accuracy reached 84.6 %; sensitivity-69 %, specificity-88.8 %, PPV-67 %, and NPV-91.1 %. Across all three steps, the early Quick Inventory of Depressive Symptomatology-Self-Report (QIDS-SR) scores were key elements in predicting the final treatment outcome. The model also identified key sociodemographic factors that predicted treatment remission at different steps.
LIMITATIONS: The retrospective design, lack of replication in an independent dataset, and the use of "a complete case analysis" model in our analysis.
CONCLUSIONS: This proof-of-concept study showed that using early treatment data, multi-step temporal prediction of depressive symptom remission results in clinically useful accuracy rates. Whether these predictive models are generalizable deserves further study.
Keywords
Humans, Depressive Disorder, Major, Artificial Intelligence, Retrospective Studies, Antidepressive Agents, Treatment Outcome, Machine Learning, Citalopram
Included in
Medical Sciences Commons, Medical Specialties Commons, Mental and Social Health Commons, Psychiatry and Psychology Commons, Psychology Commons
Comments
Supplementary Materials
PMID: 36584711