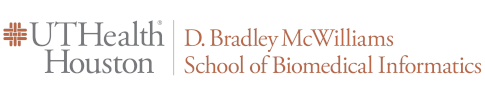
Dissertations & Theses (Open Access)
Graduation Date
Fall 12-18-2015
Degree Name
Doctor of Philosophy (PhD)
School Name
The University of Texas School of Biomedical Informatics at Houston
Advisory Committee
Jiajie Zhang, PhD
Abstract
Objective: The objective of this work is to develop process mining techniques for analysing Electronic Health Record (EHR) events in order to uncover factors contributing to the event, and understanding deviations in the process. We have outlined a method for combining data mining with expert review to model the EHR process and develop automated algorithms that can be used to detect potential deviations for a defined process.
Introduction: To analyse EHR events meaningfully, process mining can be applied to distil structured process description from a set of real executions. Process mining can be applied for 1) Discovery, 2) Conformance, and 3) Enhancement of processes. This can be used for improving efficiency and safety in the process. Extending process mining to EHR system use, user activity can be analysed to model EHR use behaviour and detect deviations from expected use. Understanding these behaviours could be used to optimize systems through redesign. Here, we explore the application of process mining of medication revisions in an EHR.
Methods: We first apply exploratory data analysis (EDA) of medication revisions (i.e. instances of altering a previous medication order) in EHR data to understand the occurrence of revision in the data. Data was retrieved from 6 U.S. ambulatory clinics, and 35,833 medication revision events were analysed. To add domain knowledge to the EDA, physicians manually reviewed a subset of events (n=100) to identify probable cause for these revisions. From the resulting causes, a categorization scheme was developed and fault trees were constructed to model the medication revision process. Additionally, from access pattern of EHR elements used in the expert review, an algorithm for automated detection of revisions was developed. Sensitivity and specificity were calculated for the algorithm used to categorize an order as a revision event.
Results: Revisions were classified into 5 categories - Cancel, Discontinue, Duplicate, Update, and Wrong Medication. 55% of the revisions were used as system workarounds to discontinue/update medications. The process model indicated that system issues were most prevalent, including problems in data entry and item selection. An automated algorithm was developed to categorize a medication order as a revision event. Given prevalence of 1.1%, the algorithm performed with 66% sensitivity, 85% accuracy and PPV of 4.8%.
Discussion: EHR medication events were process mined by applying both data mining and domain knowledge. For the majority of cases, medication revisions are used as system workarounds. The fault tree analysis also suggests a common cause of these alterations is system issues. Although our automated methods showed lower sensitivity because of these workarounds, they were able to classify successfully those medication revisions that did reflect errors such as Wrong Medication or Duplicate Medications.
Conclusion: Process mining was applied to medication revisions and was shown to detect revision events in the data. The process model uncovers factors responsible for revision events and can be used for improving EHR use. The detection algorithm can be useful in real-time monitoring. The vision is to develop monitoring tool for EHR similar to flight recorders and antivirus software.
Recommended Citation
Rogith, Deevakar, "Process Mining of Medication Revisions in Electronic Health Records" (2015). Dissertations & Theses (Open Access). 32.
https://digitalcommons.library.tmc.edu/uthshis_dissertations/32
Keywords
process mining, data mining, process analysis, process modelling, medication revisions, fault tree analysis