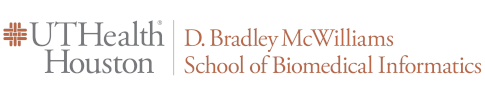
Dissertations & Theses (Open Access)
Graduation Date
12-2017
Degree Name
Doctor of Philosophy (PhD)
School Name
The University of Texas School of Biomedical Informatics at Houston
Advisory Committee
Susan H. Fenton, PhD
Abstract
Background and Objectives: The population health care delivery model uses phenotype algorithms in the electronic health record (EHR) system to identify patient cohorts targeted for clinical interventions such as laboratory tests, and procedures. The standard terminology used to identify disease cohorts may contribute to significant variation in error rates for patient inclusion or exclusion. The United States requires EHR systems to support two diagnosis terminologies, the International Classification of Disease (ICD) and the Systematized Nomenclature of Medicine (SNOMED). Terminology mapping enables the retrieval of diagnosis data using either terminology. There are no standards of practice by which to evaluate and report the operational characteristics of ICD and SNOMED value sets used to select patient groups for population health interventions. Establishing a best practice for terminology selection is a step forward in ensuring that the right patients receive the right intervention at the right time. The research question is, “How does the diagnosis retrieval terminology (ICD vs SNOMED) and terminology map maintenance impact population health cohorts?” Aim 1 and 2 explore this question, and Aim 3 informs practice and policy for population health programs.
Methods
Aim 1: Quantify impact of terminology choice (ICD vs SNOMED)
ICD and SNOMED phenotype algorithms for diabetes, chronic kidney disease (CKD), and heart failure were developed using matched sets of codes from the Value Set Authority Center. The performance of the diagnosis-only phenotypes was compared to published reference standard that included diagnosis codes, laboratory results, procedures, and medications.
Aim 2: Measure terminology maintenance impact on SNOMED cohorts
For each disease state, the performance of a single SNOMED algorithm before and after terminology updates was evaluated in comparison to a reference standard to identify and quantify cohort changes introduced by terminology maintenance.
Aim 3: Recommend methods for improving population health interventions
The socio-technical model for studying health information technology was used to inform best practice for the use of population health interventions.
Results
Aim 1: ICD-10 value sets had better sensitivity than SNOMED for diabetes (.829, .662) and CKD (.242, .225) (N=201,713, p
Aim 2: Following terminology maintenance the SNOMED algorithm for diabetes increased in sensitivity from (.662 to .683 (p
Aim 3: Based on observed social and technical challenges to population health programs, including and in addition to the development and measurement of phenotypes, a practical method was proposed for population health intervention development and reporting.
Recommended Citation
Berkovich, Barbara A., "Impact of Terminology Mapping on Population Health Cohorts IMPaCt" (2017). Dissertations & Theses (Open Access). 40.
https://digitalcommons.library.tmc.edu/uthshis_dissertations/40
Keywords
Electronic Health Records, terminology mapping, International Classification of Disease, SNOMED