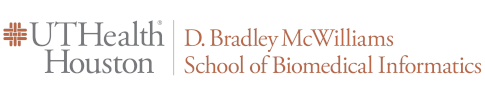
Dissertations & Theses (Open Access)
Graduation Date
2018
Degree Name
Doctor of Philosophy (PhD)
School Name
The University of Texas School of Biomedical Informatics at Houston
Advisory Committee
Trevor Cohen, MBChB, PhD
Abstract
Prior work in causal modeling has focused primarily on learning graph structures and parameters to model data generating processes from observational or experimental data, while the focus of the literature-based discovery paradigm was to identify novel therapeutic hypotheses in publicly available knowledge. The critical contribution of this dissertation is to refashion the literature-based discovery paradigm as a means to populate causal models with relevant covariates to abet causal inference. In particular, this dissertation describes a generalizable framework for mapping from causal propositions in the literature to subgraphs populated by instantiated variables that reflect observational data. The observational data are those derived from electronic health records. The purpose of causal inference is to detect adverse drug event signals. The Principle of the Common Cause is exploited as a heuristic for a defeasible practical logic. The fundamental intuition is that improbable co-occurrences can be “explained away” with reference to a common cause, or confounder. Semantic constraints in literature-based discovery can be leveraged to identify such covariates. Further, the asymmetric semantic constraints of causal propositions map directly to the topology of causal graphs as directed edges. The hypothesis is that causal models conditioned on sets of such covariates will improve upon the performance of purely statistical techniques for detecting adverse drug event signals. By improving upon previous work in purely EHR-based pharmacovigilance, these results establish the utility of this scalable approach to automated causal inference.
Recommended Citation
Malec, Scott, "Using the Literature to Identify Confounders" (2018). Dissertations & Theses (Open Access). 41.
https://digitalcommons.library.tmc.edu/uthshis_dissertations/41
Keywords
Causal modeling, literature-based discovery, EHR-derived data, pharmacovigilance