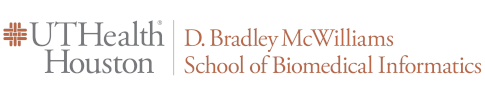
Dissertations & Theses (Open Access)
Graduation Date
Spring 5-1-2020
Degree Name
Doctor of Philosophy (PhD)
School Name
The University of Texas School of Biomedical Informatics at Houston
Advisory Committee
Hua Xu, PhD
Abstract
Unprecedented amounts of data have been generated in the biomedical domain, and the bottleneck for biomedical research has shifted from data generation to data management, interpretation, and communication. Therefore, it is highly desirable to develop systems to assist in text generation from biomedical data, which will greatly improve the dissemination of scientific findings. However, very few studies have investigated issues of data-to-text generation in the biomedical domain. Here I present a systematic study for generating descriptive text from tables in randomized clinical trials (RCT) articles, which includes: (1) an information model for representing RCT tables; (2) annotated corpora containing pairs of RCT table and descriptive text, and labeled structural and semantic information of RCT tables; (3) methods for recognizing structural and semantic information of RCT tables; (4) methods for generating text from RCT tables, evaluated by a user study on three aspects: relevance, grammatical quality, and matching. The proposed hybrid text generation method achieved a low bilingual evaluation understudy (BLEU) score of 5.69; but human review achieved scores of 9.3, 9.9 and 9.3 for relevance, grammatical quality and matching, respectively, which are comparable to review of original human-written text. To the best of our knowledge, this is the first study to generate text from scientific tables in the biomedical domain. The proposed information model, labeled corpora and developed methods for recognizing tables and generating descriptive text could also facilitate other biomedical and informatics research and applications.
Recommended Citation
Wei, Qiang, "Table-to-Text: Generating Descriptive Text for Scientific Tables from Randomized Controlled Trials" (2020). Dissertations & Theses (Open Access). 48.
https://digitalcommons.library.tmc.edu/uthshis_dissertations/48
Keywords
Natural language generation, randomized controlled trial, information extraction from table, deep learning, named entity recognition, parsing of table structure, information model, data to text, language model