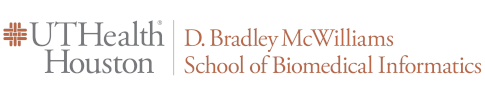
Dissertations & Theses (Open Access)
Graduation Date
Fall 2021
Degree Name
Doctor of Philosophy (PhD)
School Name
The University of Texas School of Biomedical Informatics at Houston
Advisory Committee
Kirk Roberts, PhD
Abstract
Medicine is undergoing a technological revolution. Understanding human health from clinical data has major challenges from technical and practical perspectives, thus prompting methods that understand large, complex, and noisy data. These methods are particularly necessary for natural language data from clinical narratives/notes, which contain some of the richest information on a patient. Meanwhile, deep neural networks have achieved superior performance in a wide variety of natural language processing (NLP) tasks because of their capacity to encode meaningful but abstract representations and learn the entire task end-to-end. In this thesis, I investigate representation learning of clinical narratives with deep neural networks through a number of tasks ranging from clinical concept extraction, clinical note modeling, and patient-level language representation. I present methods utilizing representation learning with neural networks to support understanding of clinical text documents.
I first introduce the notion of representation learning from natural language processing and patient data modeling. Then, I investigate word-level representation learning to improve clinical concept extraction from clinical notes. I present two works on learning word representations and evaluate them to extract important concepts from clinical notes. The first study focuses on cancer-related information, and the second study evaluates shared-task data. The aims of these two studies are to automatically extract important entities from clinical notes. Next, I present a series of deep neural networks to encode hierarchical, longitudinal, and contextual information for modeling a series of clinical notes. I also evaluate the models by predicting clinical outcomes of interest, including mortality, length of stay, and phenotype predictions. Finally, I propose a novel representation learning architecture to develop a generalized and transferable language representation at the patient level. I also identify pre-training tasks appropriate for constructing a generalizable language representation. The main focus is to improve predictive performance of phenotypes with limited data, a challenging task due to a lack of data.
Overall, this dissertation addresses issues in natural language processing for medicine, including clinical text classification and modeling. These studies show major barriers to understanding large-scale clinical notes. It is believed that developing deep representation learning methods for distilling enormous amounts of heterogeneous data into patient-level language representations will improve evidence-based clinical understanding. The approach to solving these issues by learning representations could be used across clinical applications despite noisy data. I conclude that considering different linguistic components in natural language and sequential information between clinical events is important. Such results have implications beyond the immediate context of predictions and further suggest future directions for clinical machine learning research to improve clinical outcomes. This could be a starting point for future phenotyping methods based on natural language processing that construct patient-level language representations to improve clinical predictions. While significant progress has been made, many open questions remain, so I will highlight a few works to demonstrate promising directions.
Recommended Citation
Si, Yuqi, "Enhance Representation Learning of Clinical Narrative with Neural Networks for Clinical Predictive Modeling" (2021). Dissertations & Theses (Open Access). 55.
https://digitalcommons.library.tmc.edu/uthshis_dissertations/55
Keywords
Natural language processing, Hierarchical Convolutional Neural Network, large-scale clinical notes representation, Hierarchical Attention Network, Bi-LSTMs
Comments
This dissertation has been published in the following journals:
1. Wu S, Roberts K, Datta S, Du J, Ji Z, Si Y, Soni S, Wang Q, Wei Q, Xiang Y, Zhao B, Xu H. Deep learning in clinical natural language processing: a methodical review. J Am Med Inform Assoc. 2020 Mar 1;27(3):457-470. doi: 10.1093/jamia/ocz200. PMID: 31794016; PMCID: PMC7025365.
2., Si Y, Wang J, Xu H, Roberts K. Enhancing clinical concept extraction with contextual embeddings. J Am Med Inform Assoc. 2019 Nov 1;26(11):1297-1304. doi: 10.1093/jamia/ocz096. PMID: 31265066; PMCID: PMC6798561.
3. Datta S, Si Y, Rodriguez L, Shooshan SE, Demner-Fushman D, Roberts K. Understanding spatial language in radiology: Representation framework, annotation, and spatial relation extraction from chest X-ray reports using deep learning. J Biomed Inform. 2020 Aug;108:103473. doi: 10.1016/j.jbi.2020.103473. Epub 2020 Jun 18. PMID: 32562898; PMCID: PMC7807990.
4. Si Y, Roberts K. A Frame-Based NLP System for Cancer-Related Information Extraction. AMIA Annu Symp Proc. 2018 Dec 5;2018:1524-1533. PMID: 30815198; PMCID: PMC6371330.
5. Si Y, Roberts K. Deep Patient Representation of Clinical Notes via Multi-Task Learning for Mortality Prediction. AMIA Jt Summits Transl Sci Proc. 2019 May 6;2019:779-788. PMID: 31259035; PMCID: PMC6568068.
6. Si Y, Bernstam EV, Roberts K. Generalized and transferable patient language representation for phenotyping with limited data. J Biomed Inform. 2021 Apr;116:103726. doi: 10.1016/j.jbi.2021.103726. Epub 2021 Mar 9. PMID: 33711541.