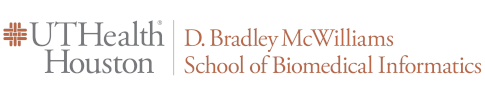
Faculty, Staff and Student Publications
Publication Date
3-14-2025
Journal
Medicine
Abstract
BACKGROUND: Scalable identification of patients with post-acute sequelae of COVID-19 (PASC) is challenging due to a lack of reproducible precision phenotyping algorithms, which has led to suboptimal accuracy, demographic biases, and underestimation of the PASC.
METHODS: In a retrospective case-control study, we developed a precision phenotyping algorithm for identifying cohorts of patients with PASC. We used longitudinal electronic health records data from over 295,000 patients from 14 hospitals and 20 community health centers in Massachusetts. The algorithm employs an attention mechanism to simultaneously exclude sequelae that prior conditions can explain and include infection-associated chronic conditions. We performed independent chart reviews to tune and validate the algorithm.
FINDINGS: The PASC phenotyping algorithm improves precision and prevalence estimation and reduces bias in identifying PASC cohorts compared to the ICD-10-CM code U09.9. The algorithm identified a cohort of over 24,000 patients with 79.9% precision. Our estimated prevalence of PASC was 22.8%, which is close to the national estimates for the region. We also provide in-depth analyses, encompassing identified lingering effects by organ, comorbidity profiles, and temporal differences in the risk of PASC.
CONCLUSIONS: PASC precision phenotyping boasts superior precision and prevalence estimation while exhibiting less bias in identifying patients with PASC. The cohort derived from this algorithm will serve as a springboard for delving into the genetic, metabolomic, and clinical intricacies of PASC, surmounting the constraints of prior PASC cohort studies.
FUNDING: This research was funded by the US National Institute of Allergy and Infectious Diseases (NIAID).
Keywords
Humans, COVID-19, Phenotype, Retrospective Studies, Algorithms, Massachusetts, Post-Acute COVID-19 Syndrome, Female, Male, Case-Control Studies, Middle Aged, Electronic Health Records, SARS-CoV-2, Adult, Prevalence, Aged
DOI
10.1016/j.medj.2024.10.009
PMID
39520983
PMCID
PMC11911085
PubMedCentral® Posted Date
3-17-2025
PubMedCentral® Full Text Version
Author MSS
Published Open-Access
yes
Graphical Abstract
Included in
Bioinformatics Commons, Biomedical Informatics Commons, COVID-19 Commons, Data Science Commons, Diseases Commons, Epidemiology Commons, Internal Medicine Commons