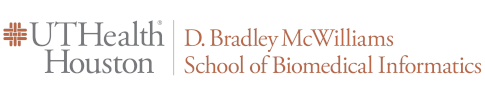
Faculty, Staff and Student Publications
Publication Date
4-1-2023
Journal
Heliyon
Abstract
Triple negative breast cancers (TNBCs) are tumors with a poor treatment response and prognosis. In this study, we propose a new approach, candidate extraction from convolutional neural network (CNN) elements (CECE), for discovery of biomarkers for TNBCs. We used the GSE96058 and GSE81538 datasets to build a CNN model to classify TNBCs and non-TNBCs and used the model to make TNBC predictions for two additional datasets, the cancer genome atlas (TCGA) breast cancer RNA sequencing data and the data from Fudan University Shanghai Cancer Center (FUSCC). Using correctly predicted TNBCs from the GSE96058 and TCGA datasets, we calculated saliency maps for these subjects and extracted the genes that the CNN model used to separate TNBCs from non-TNBCs. Among the TNBC signature patterns that the CNN models learned from the training data, we found a set of 21 genes that can classify TNBCs into two major classes, or CECE subtypes, with distinct overall survival rates (
Comments
Supplementary Materials
PMID: 37025902