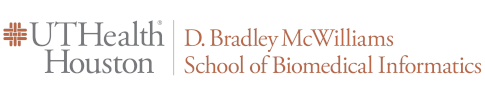
Student and Faculty Publications
Publication Date
1-1-2023
Journal
AMIA Summits on Translational Science Proceedings
Abstract
The Unified Medical Language System (UMLS), a large repository of biomedical vocabularies, has been used for supporting various biomedical applications. Ensuring the quality of the UMLS is critical to maintain both the accuracy of its content and the reliability of downstream applications. In this work, we present a Graph Convolutional Network (GCN)-based approach to identify misaligned synonymous terms organized under different UMLS concepts. We used synonymous terms grouped under the same concept as positive samples and top lexically similar terms as negative samples to train the GCN model. We applied the model to a test set and suggested those negative samples predicted to be synonymous as potentially misaligned synonymous terms. A total of 147,625 suggestions were made. A human expert evaluated 100 randomly selected suggestions and agreed with 60 of them. The results indicate that our GCN-based approach shows promise to help improve the synonymy grouping in the UMLS.
Keywords
Humans, Unified Medical Language System, Reproducibility of Results
Comments
PMID: 38222357