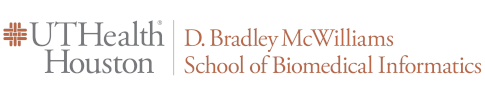
Faculty, Staff and Student Publications
Publication Date
1-1-2023
Journal
IEEE Transactions on Biomedical Engineering
Abstract
Parkinson's disease (PD) is the second most prevalent neurodegenerative disease disorder in the world. A prompt diagnosis would enable clinical trials for disease-modifying neuroprotective therapies. Recent research efforts have unveiled imaging and blood markers that have the potential to be used to identify PD patients promptly, however, the idiopathic nature of PD makes these tests very hard to scale to the general population. To this end, we need an easily deployable tool that would enable screening for PD signs in the general population. In this work, we propose a new set of features based on keystroke dynamics, i.e., the time required to press and release keyboard keys during typing, and used to detect PD in an ecologically valid data acquisition setup at the subject's homes, without requiring any pre-defined task. We compare and contrast existing models presented in the literature and present a new model that combines a new type of keystroke dynamics signal representation using hold time and flight time series as a function of key types and asymmetry in the time series using a convolutional neural network. We show how this model achieves an Area Under the Receiving Operating Characteristic curve ranging from 0.80 to 0.83 on a dataset of subjects who actively interacted with their computers for at least 5 months and positively compares against other state-of-the-art approaches previously tested on keystroke dynamics data acquired with mechanical keyboards.
Keywords
Humans, Parkinson Disease, Neurodegenerative Diseases, Benchmarking, Computers, Neural Networks, Computer
DOI
10.1109/TBME.2022.3187309
PMID
35767495
PMCID
PMC9904385
PubMedCentral® Posted Date
January 2024
PubMedCentral® Full Text Version
Author MSS
Comments
PMID: 35767495