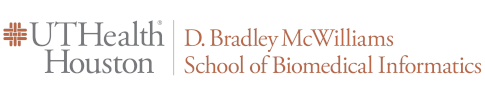
Journal Articles
Publication Date
9-27-2022
Journal
Scientific Reports
Abstract
Computational models have been successful in predicting drug sensitivity in cancer cell line data, creating an opportunity to guide precision medicine. However, translating these models to tumors remains challenging. We propose a new transfer learning workflow that transfers drug sensitivity predicting models from large-scale cancer cell lines to both tumors and patient derived xenografts based on molecular pathways derived from genomic features. We further compute feature importance to identify pathways most important to drug response prediction. We obtained good performance on tumors (AUROC = 0.77) and patient derived xenografts from triple negative breast cancers (RMSE = 0.11). Using feature importance, we highlight the association between ER-Golgi trafficking pathway in everolimus sensitivity within breast cancer patients and the role of class II histone deacetylases and interlukine-12 in response to drugs for triple-negative breast cancer. Pathway information support transfer of drug response prediction models from cell lines to tumors and can provide biological interpretation underlying the predictions, serving as a steppingstone towards usage in clinical setting.
Keywords
Cell Line, Cell Line, Tumor, Everolimus, Heterografts, Histone Deacetylases, Humans, Machine Learning, Triple Negative Breast Neoplasms
Included in
Biomedical Informatics Commons, Medical Education Commons, Medical Sciences Commons, Oncology Commons
Comments
Supplementary Materials
PMID: 36168036