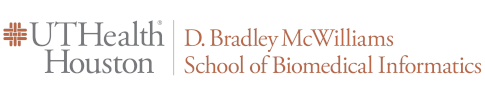
Faculty, Staff and Student Publications
Publication Date
1-1-2024
Journal
AMIA Summits on Translational Science Proceedings
Abstract
The results of clinical trials are a valuable source of evidence for researchers, policy makers, and healthcare professionals. However, online trial registries do not always contain links to the publications that report on their results, instead requiring a time-consuming manual search. Here, we explored the application of pre-trained transformer-based language models to automatically identify result-reporting publications of cancer clinical trials by computing dense vectors and performing semantic search. Models were fine-tuned on text data from trial registry fields and article metadata using a contrastive learning approach. The best performing model was PubMedBERT, which achieved a mean average precision of 0.592 and ranked 70.3% of a trial's publications in the top 5 results when tested on the holdout test trials. Our results suggest that semantic search using embeddings from transformer models may be an effective approach to the task of linking trials to their publications.
Comments
PMID: 38827077