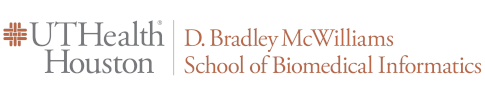
Faculty, Staff and Student Publications
Publication Date
1-1-2022
Journal
Brain Communications
Abstract
Measuring cognitive function is essential for characterizing brain health and tracking cognitive decline in Alzheimer's Disease and other neurodegenerative conditions. Current tools to accurately evaluate cognitive impairment typically rely on a battery of questionnaires administered during clinical visits which is essential for the acquisition of repeated measurements in longitudinal studies. Previous studies have shown that the remote data collection of passively monitored daily interaction with personal digital devices can measure motor signs in the early stages of synucleinopathies, as well as facilitate longitudinal patient assessment in the real-world scenario with high patient compliance. This was achieved by the automatic discovery of patterns in the time series of keystroke dynamics, i.e. the time required to press and release keys, by machine learning algorithms. In this work, our hypothesis is that the typing patterns generated from user-device interaction may reflect relevant features of the effects of cognitive impairment caused by neurodegeneration. We use machine learning algorithms to estimate cognitive performance through the analysis of keystroke dynamic patterns that were extracted from mechanical and touchscreen keyboard use in a dataset of cognitively normal (
DOI
10.1093/braincomms/fcac194
PMID
35950091
PMCID
PMC9356723
PubMedCentral® Posted Date
7-28-2022
PubMedCentral® Full Text Version
Post-print
Published Open-Access
yes
Included in
Behavior and Behavior Mechanisms Commons, Bioinformatics Commons, Biomedical Informatics Commons, Data Science Commons, Health Information Technology Commons, Neurology Commons