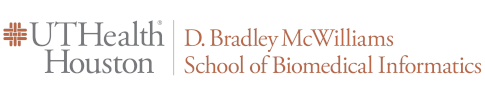
Faculty, Staff and Student Publications
Publication Date
1-25-2022
Journal
Healthcare
Abstract
BACKGROUND: An increase in opioid use has led to an opioid crisis during the last decade, leading to declarations of a public health emergency. In response to this call, the Houston Emergency Opioid Engagement System (HEROES) was established and created an emergency access pathway into long-term recovery for individuals with an opioid use disorder. A major contributor to the success of the program is retention of the enrolled individuals in the program.
METHODS: We have identified an increase in dropout from the program after 90 and 120 days. Based on more than 700 program participants, we developed a machine learning approach to predict the individualized risk for dropping out of the program.
RESULTS: Our model achieved sensitivity of 0.81 and specificity of 0.65 for dropout at 90 days and improved the performance to sensitivity of 0.86 and specificity of 0.66 for 120 days. Additionally, we identified individual risk factors for dropout, including previous overdose and relapse and improvement in reported quality of life.
CONCLUSIONS: Our informatics approach provides insight into an area where programs may allocate additional resources in order to retain high-risk individuals and increase the chances of success in recovery.
Keywords
opioid use disorder, machine learning, predictive modeling, treatment, substance use disorder
DOI
10.3390/healthcare10020223
PMID
35206838
PMCID
PMC8871589
PubMedCentral® Posted Date
1-25-2022
PubMedCentral® Full Text Version
Post-print
Published Open-Access
yes
Included in
Bioinformatics Commons, Biomedical Informatics Commons, Data Science Commons, Public Health Commons, Social and Behavioral Sciences Commons, Substance Abuse and Addiction Commons