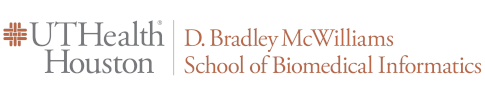
Faculty, Staff and Student Publications
Publication Date
2-16-2024
Journal
Journal of the American Medical Informatics Association
Abstract
OBJECTIVE: The HIV epidemic remains a significant public health issue in the United States. HIV risk prediction models could be beneficial for reducing HIV transmission by helping clinicians identify patients at high risk for infection and refer them for testing. This would facilitate initiation on treatment for those unaware of their status and pre-exposure prophylaxis for those uninfected but at high risk. Existing HIV risk prediction algorithms rely on manual construction of features and are limited in their application across diverse electronic health record systems. Furthermore, the accuracy of these models in predicting HIV in females has thus far been limited.
MATERIALS AND METHODS: We devised a pipeline for automatic construction of prediction models based on automatic feature engineering to predict HIV risk and tested our pipeline on a local electronic health records system and a national claims data. We also compared the performance of general models to female-specific models.
RESULTS: Our models obtain similarly good performance on both health record datasets despite difference in represented populations and data availability (AUC = 0.87). Furthermore, our general models obtain good performance on females but are also improved by constructing female-specific models (AUC between 0.81 and 0.86 across datasets).
DISCUSSION AND CONCLUSIONS: We demonstrated that flexible construction of prediction models performs well on HIV risk prediction across diverse health records systems and perform as well in predicting HIV risk in females, making deployment of such models into existing health care systems tangible.
Keywords
Humans, Female, United States, Electronic Health Records, Software, Algorithms, Machine Learning, HIV Infections, HIV, risk prediction, electronic health records, HIV prevention, predictive modeling
DOI
10.1093/jamia/ocad217
PMID
37990631
PMCID
PMC10873846
PubMedCentral® Posted Date
11-21-2023
PubMedCentral® Full Text Version
Post-print
Published Open-Access
yes