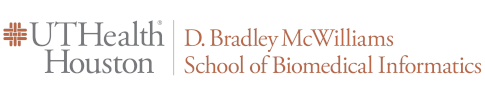
Faculty, Staff and Student Publications
Publication Date
8-15-2022
Journal
Nature Communications
Abstract
Advanced computer vision technology can provide near real-time home monitoring to support "aging in place" by detecting falls and symptoms related to seizures and stroke. Affordable webcams, together with cloud computing services (to run machine learning algorithms), can potentially bring significant social benefits. However, it has not been deployed in practice because of privacy concerns. In this paper, we propose a strategy that uses homomorphic encryption to resolve this dilemma, which guarantees information confidentiality while retaining action detection. Our protocol for secure inference can distinguish falls from activities of daily living with 86.21% sensitivity and 99.14% specificity, with an average inference latency of 1.2 seconds and 2.4 seconds on real-world test datasets using small and large neural nets, respectively. We show that our method enables a 613x speedup over the latency-optimized LoLa and achieves an average of 3.1x throughput increase in secure inference compared to the throughput-optimized nGraph-HE2.
Keywords
Activities of Daily Living, Algorithms, Cloud Computing, Computer Security, Humans, Neural Networks, Computer, Pattern Recognition, Automated
DOI
10.1038/s41467-022-32168-5
PMID
35970834
PMCID
PMC9378731
PubMedCentral® Posted Date
8-15-2022
PubMedCentral® Full Text Version
Post-print
Published Open-Access
yes
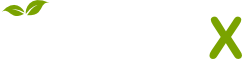
- Citations
- Citation Indexes: 27
- Usage
- Downloads: 46
- Abstract Views: 1
- Captures
- Readers: 37
- Mentions
- Blog Mentions: 1
- News Mentions: 3
Included in
Bioinformatics Commons, Biomedical Informatics Commons, Computer Sciences Commons, Data Science Commons, Geriatrics Commons, Neurology Commons, Neurosciences Commons