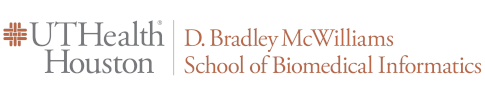
Faculty, Staff and Student Publications
Publication Date
3-1-2022
Journal
Journal of Clinical Periodontology
Abstract
AIM: The goal was to use a deep convolutional neural network to measure the radiographic alveolar bone level to aid periodontal diagnosis.
MATERIALS AND METHODS: A deep learning (DL) model was developed by integrating three segmentation networks (bone area, tooth, cemento-enamel junction) and image analysis to measure the radiographic bone level and assign radiographic bone loss (RBL) stages. The percentage of RBL was calculated to determine the stage of RBL for each tooth. A provisional periodontal diagnosis was assigned using the 2018 periodontitis classification. RBL percentage, staging, and presumptive diagnosis were compared with the measurements and diagnoses made by the independent examiners.
RESULTS: The average Dice Similarity Coefficient (DSC) for segmentation was over 0.91. There was no significant difference in the RBL percentage measurements determined by DL and examiners (
CONCLUSIONS: The proposed DL model provides reliable RBL measurements and image-based periodontal diagnosis using periapical radiographic images. However, this model has to be further optimized and validated by a larger number of images to facilitate its application.
Keywords
Deep Learning, Humans, Image Processing, Computer-Assisted, Neural Networks, Computer, Periodontitis, diagnosis, deep learning, periodontal diseases, radiographic image interpretation
DOI
10.1111/jcpe.13574
PMID
34879437
PMCID
PMC9026777
PubMedCentral® Posted Date
4-22-2022
PubMedCentral® Full Text Version
Author MSS
Published Open-Access
yes
Included in
Bioinformatics Commons, Biomedical Informatics Commons, Community Health and Preventive Medicine Commons, Data Science Commons, Periodontics and Periodontology Commons