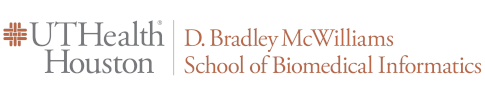
Faculty, Staff and Student Publications
Publication Date
10-16-2022
Journal
BMC Medical Informatics and Decision Making
Abstract
OBJECTIVES: This paper developed federated solutions based on two approximation algorithms to achieve federated generalized linear mixed effect models (GLMM). The paper also proposed a solution for numerical errors and singularity issues. And showed the two proposed methods can perform well in revealing the significance of parameter in distributed datasets, comparing to a centralized GLMM algorithm from R package ('lme4') as the baseline model.
METHODS: The log-likelihood function of GLMM is approximated by two numerical methods (Laplace approximation and Gaussian Hermite approximation, abbreviated as LA and GH), which supports federated decomposition of GLMM to bring computation to data. To solve the numerical errors and singularity issues, the loss-less estimation of log-sum-exponential trick and the adaptive regularization strategy was used to tackle the problems caused by federated settings.
RESULTS: Our proposed method can handle GLMM to accommodate hierarchical data with multiple non-independent levels of observations in a federated setting. The experiment results demonstrate comparable (LA) and superior (GH) performances with simulated and real-world data.
CONCLUSION: We modified and compared federated GLMMs with different approximations, which can support researchers in analyzing versatile biomedical data to accommodate mixed effects and address non-independence due to hierarchical structures (i.e., institutes, region, country, etc.).
Keywords
Algorithms, Computer Simulation, Humans, Likelihood Functions, Linear Models, Research Design, GLMM, Federated learning, Mixed effects, Laplace approximation, Gauss–Hermite approximation
DOI
10.1186/s12911-022-02014-1
PMID
36244993
PMCID
PMC9569919
PubMedCentral® Posted Date
10-16-2022
PubMedCentral® Full Text Version
Post-print
Published Open-Access
yes
Included in
Bioinformatics Commons, Biomedical Informatics Commons, Data Science Commons, Genetic Phenomena Commons, Medical Genetics Commons