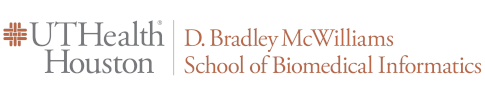
Faculty, Staff and Student Publications
Publication Date
5-13-2022
Journal
Bioinformatics
Abstract
MOTIVATION: Evaluating the blood-brain barrier (BBB) permeability of drug molecules is a critical step in brain drug development. Traditional methods for the evaluation require complicated in vitro or in vivo testing. Alternatively, in silico predictions based on machine learning have proved to be a cost-efficient way to complement the in vitro and in vivo methods. However, the performance of the established models has been limited by their incapability of dealing with the interactions between drugs and proteins, which play an important role in the mechanism behind the BBB penetrating behaviors. To address this limitation, we employed the relational graph convolutional network (RGCN) to handle the drug-protein interactions as well as the properties of each individual drug.
RESULTS: The RGCN model achieved an overall accuracy of 0.872, an area under the receiver operating characteristic (AUROC) of 0.919 and an area under the precision-recall curve (AUPRC) of 0.838 for the testing dataset with the drug-protein interactions and the Mordred descriptors as the input. Introducing drug-drug similarity to connect structurally similar drugs in the data graph further improved the testing results, giving an overall accuracy of 0.876, an AUROC of 0.926 and an AUPRC of 0.865. In particular, the RGCN model was found to greatly outperform the LightGBM base model when evaluated with the drugs whose BBB penetration was dependent on drug-protein interactions. Our model is expected to provide high-confidence predictions of BBB permeability for drug prioritization in the experimental screening of BBB-penetrating drugs.
AVAILABILITY AND IMPLEMENTATION: The data and the codes are freely available at https://github.com/dingyan20/BBB-Penetration-Prediction.
Keywords
Biological Transport, Blood-Brain Barrier, Brain, Machine Learning, Permeability, Proteins
DOI
10.1093/bioinformatics/btac211
PMID
35561199
PMCID
PMC9113341
PubMedCentral® Posted Date
4-7-2022
PubMedCentral® Full Text Version
Post-print
Published Open-Access
yes
Supplementary Information