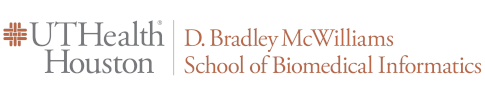
Faculty, Staff and Student Publications
Publication Date
7-1-2023
Journal
Journal of Medical Imaging
Abstract
PURPOSE: In women with biopsy-proven breast cancer, histologically normal areas of the parenchyma have shown molecular similarity to the tumor, supporting a potential cancer field effect. The purpose of this work was to investigate relationships of human-engineered radiomic and deep learning features between regions across the breast in mammographic parenchymal patterns and specimen radiographs.
APPROACH: This study included mammograms from 74 patients with at least 1 identified malignant tumor, of whom 32 also possessed intraoperative radiographs of mastectomy specimens. Mammograms were acquired with a Hologic system and specimen radiographs were acquired with a Fujifilm imaging system. All images were retrospectively collected under an Institutional Review Board-approved protocol. Regions of interest (ROI) of
RESULTS: Statistically significant correlations in select subgroups of features with tumor, near to the tumor, and far from the tumor ROI regions were identified in both mammograms and specimen radiographs. Intensity-based features were found to show significant correlations with ROI regions across both modalities.
CONCLUSIONS: Results support our hypothesis of a potential cancer field effect, accessible radiographically, across tumor and non-tumor regions, thus indicating the potential for computerized analysis of mammographic parenchymal patterns to predict breast cancer risk.
DOI
10.1117/1.JMI.10.4.044501
PMID
37426053
PMCID
PMC10329416
PubMedCentral® Posted Date
7-10-2023
PubMedCentral® Full Text Version
Post-print
Published Open-Access
yes
Included in
Bioinformatics Commons, Biomedical Informatics Commons, Obstetrics and Gynecology Commons, Oncology Commons