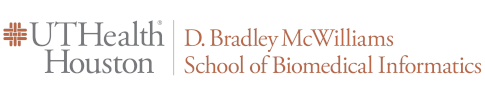
Faculty, Staff and Student Publications
Publication Date
5-24-2024
Journal
BMC Medical Imaing
Abstract
BACKGROUND: Lung cancer is the second most common cancer worldwide, with over two million new cases per year. Early identification would allow healthcare practitioners to handle it more effectively. The advancement of computer-aided detection systems significantly impacted clinical analysis and decision-making on human disease. Towards this, machine learning and deep learning techniques are successfully being applied. Due to several advantages, transfer learning has become popular for disease detection based on image data.
METHODS: In this work, we build a novel transfer learning model (VER-Net) by stacking three different transfer learning models to detect lung cancer using lung CT scan images. The model is trained to map the CT scan images with four lung cancer classes. Various measures, such as image preprocessing, data augmentation, and hyperparameter tuning, are taken to improve the efficacy of VER-Net. All the models are trained and evaluated using multiclass classifications chest CT images.
RESULTS: The experimental results confirm that VER-Net outperformed the other eight transfer learning models compared with. VER-Net scored 91%, 92%, 91%, and 91.3% when tested for accuracy, precision, recall, and F1-score, respectively. Compared to the state-of-the-art, VER-Net has better accuracy.
CONCLUSION: VER-Net is not only effectively used for lung cancer detection but may also be useful for other diseases for which CT scan images are available.
Keywords
Humans, Lung Neoplasms, Tomography, X-Ray Computed, Machine Learning, Deep Learning, Radiographic Image Interpretation, Computer-Assisted, Lung cancer detection, CT scan, Transfer learning, Image processing, Stacking, VGG19, EfficientNetB0, ResNet101
DOI
10.1186/s12880-024-01238-z
PMID
38789925
PMCID
PMC11127393
PubMedCentral® Full Text Version
Post-print
Published Open-Access
yes
Correction
Included in
Bioinformatics Commons, Biomedical Informatics Commons, Medical Sciences Commons, Oncology Commons
Comments
This article has been corrected. See BMC Med Imaging. 2024 May 31;24:128.