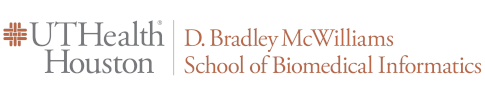
Faculty, Staff and Student Publications
Publication Date
12-20-2024
Journal
Medicine
Abstract
Purpose: To create a nomogram for accurate prognosis of patients with clear cell renal cell carcinoma (ccRCC) based on computed tomography images.
Methods: Eight hundred twenty-two ccRCC patients with contrast-enhanced computed tomography images involved in this study were collected. A rectangular region of interest surrounding the tumor was used to extract quantitative radiomics and deep-learning features, which were filtered by Cox proportional hazard regression model and least absolute shrinkage and selection operator. Then the selected features formed a fusion signature, which was assessed by Cox proportional hazard regression model method, Kaplan-Meier analysis, receiver operating characteristic curves, and concordance index (C-index) in different clinical subgroups. Finally, a nomogram constructed with this signature and clinicopathologic risk factors was assessed by C-index and survival calibration curves.
Results: The fusion signature performed better than the radiomics signature. Then we combined this signature and 2 clinicopathologic risk factors. This nomogram showed an increase of about 20% in C-index values when compared to clinical nomogram in both datasets. Its prediction probability was also in good agreement with the actual ratio.
Conclusion: The proposed fusion nomogram provided a noninvasive and easy-to-use model for survival prognosis of ccRCC patients in future clinical use, without the requirement to perform a detailed segmentation for radiologists.
Keywords
Humans, Carcinoma, Renal Cell, Deep Learning, Female, Male, Kidney Neoplasms, Tomography, X-Ray Computed, Middle Aged, Nomograms, Aged, Survival Analysis, Prognosis, Proportional Hazards Models, Adult, Kaplan-Meier Estimate, ROC Curve, Retrospective Studies, Risk Factors, Radiomics
DOI
10.1097/MD.0000000000040723
PMID
39705434
PMCID
PMC11666162
PubMedCentral® Posted Date
12-20-2024
PubMedCentral® Full Text Version
Post-print
Published Open-Access
yes