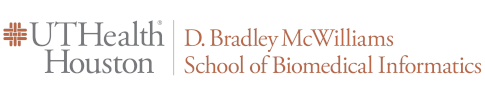
Faculty, Staff and Student Publications
Publication Date
10-8-2022
Journal
Genome Medicine
Abstract
Multiple computational approaches have been developed to improve our understanding of genetic variants. However, their ability to identify rare pathogenic variants from rare benign ones is still lacking. Using context annotations and deep learning methods, we present pathogenicity prediction models, MetaRNN and MetaRNN-indel, to help identify and prioritize rare nonsynonymous single nucleotide variants (nsSNVs) and non-frameshift insertion/deletions (nfINDELs). We use independent test sets to demonstrate that these new models outperform state-of-the-art competitors and achieve a more interpretable score distribution. Importantly, prediction scores from both models are comparable, enabling easy adoption of integrated genotype-phenotype association analysis methods. All pre-computed nsSNV scores are available at http://www.liulab.science/MetaRNN. The stand-alone program is also available at https://github.com/Chang-Li2019/MetaRNN.
Keywords
Computational Biology, Deep Learning, INDEL Mutation, Nucleotides
DOI
10.1186/s13073-022-01120-z
PMID
36209109
PMCID
PMC9548151
PubMedCentral® Posted Date
10-8-2022
PubMedCentral® Full Text Version
Post-print
Published Open-Access
yes
Included in
Bioinformatics Commons, Biomedical Informatics Commons, Genetics and Genomics Commons, Medical Genetics Commons