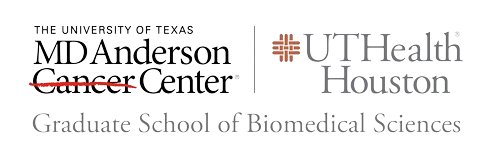
Faculty, Staff and Student Publications
Publication Date
2-28-2023
Journal
Genome Biology
Abstract
Bulk high-throughput omics data contain signals from a mixture of cell types. Recent developments of deconvolution methods facilitate cell type-specific inferences from bulk data. Our real data exploration suggests that differential expression or methylation status is often correlated among cell types. Based on this observation, we develop a novel statistical method named CeDAR to incorporate the cell type hierarchy in cell type-specific differential analyses of bulk data. Extensive simulation and real data analyses demonstrate that this approach significantly improves the accuracy and power in detecting cell type-specific differential signals compared with existing methods, especially in low-abundance cell types.
Keywords
Computer Simulation, Data Analysis, Protein Processing, Post-Translational, Cell type-specific differential analysis, Cell type hierarchy, Hierarchical Bayesian model, Microarray data analysis
DOI
i10.1186/s13059-023-02857-5
PMID
36855165
PMCID
PMC9972684
PubMedCentral® Posted Date
February 2023
PubMedCentral® Full Text Version
Post-print
Published Open-Access
yes
Included in
Bioinformatics Commons, Biomedical Informatics Commons, Medical Sciences Commons, Oncology Commons