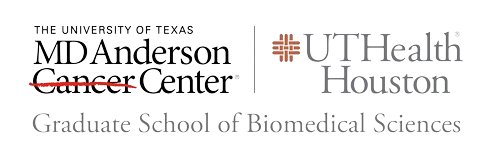
Faculty, Staff and Student Publications
Publication Date
7-31-2023
Journal
Cancers
Abstract
Head and neck squamous cell carcinoma (HNSCC), specifically in the oral cavity (oral squamous cell carcinoma, OSCC), is a common, complex cancer that significantly affects patients' quality of life. Early diagnosis typically improves prognoses yet relies on pathologist examination of histology images that exhibit high inter- and intra-observer variation. The advent of deep learning has automated this analysis, notably with object segmentation. However, techniques for automated oral dysplasia diagnosis have been limited to shape or cell stain information, without addressing the diagnostic potential in counting the number of cell layers in the oral epithelium. Our study attempts to address this gap by combining the existing U-Net and HD-Staining architectures for segmenting the oral epithelium and introducing a novel algorithm that we call Onion Peeling for counting the epithelium layer number. Experimental results show a close correlation between our algorithmic and expert manual layer counts, demonstrating the feasibility of automated layer counting. We also show the clinical relevance of oral epithelial layer number to grading oral dysplasia severity through survival analysis. Overall, our study shows that automated counting of oral epithelium layers can represent a potential addition to the digital pathology toolbox. Model generalizability and accuracy could be improved further with a larger training dataset.
Keywords
oral cavity and pharyngeal cancer, oral epithelium, histology, medical image analysis, deep learning
DOI
10.3390/cancers15153891
PMID
37568707
PMCID
PMC10416878
PubMedCentral® Posted Date
July 2023
PubMedCentral® Full Text Version
Post-print
Published Open-Access
yes
Included in
Bioinformatics Commons, Biomedical Informatics Commons, Medical Sciences Commons, Oncology Commons
Comments
Supplementary Materials
Data Availability Statement
PMID: 37568707