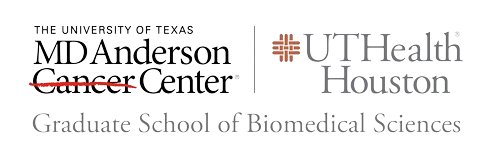
Faculty, Staff and Student Publications
Publication Date
12-1-2024
Journal
Annals of Medicine
Abstract
OBJECTIVE: Investigation of explainable deep learning methods for graph neural networks to predict HIV infections with social network information and performing domain adaptation to evaluate model transferability across different datasets.
METHODS: Network data from two cohorts of younger sexual minority men (SMM) from two U.S. cities (Chicago, IL, and Houston, TX) were collected between 2014 and 2016. Feature importance from graph attention network (GAT) models were determined using GNNExplainer. Domain adaptation was performed to examine model transferability from one city dataset to the other dataset, training with 100% of the source dataset with 30% of the target dataset and prediction on the remaining 70% from the target dataset.
RESULTS: Domain adaptation showed the ability of GAT to improve prediction over training with single city datasets. Feature importance analysis with GAT models in single city training indicated similar features across different cities, reinforcing potential application of GAT models in predicting HIV infections through domain adaptation.
CONCLUSION: GAT models can be used to address the data sparsity issue in HIV study populations. They are powerful tools for predicting individual risk of HIV that can be further explored for better understanding of HIV transmission.
Keywords
Humans, HIV Infections, Male, Neural Networks, Computer, Deep Learning, Adult, Sexual and Gender Minorities, Artificial Intelligence, Young Adult, Homosexuality, Male. Graph machine learning, explainable artificial intelligence, HIV, domain adaptation
DOI
10.1080/07853890.2024.2407063
PMID
39417227
PMCID
PMC11488171
PubMedCentral® Posted Date
October 2024
PubMedCentral® Full Text Version
Post-print
Published Open-Access
yes
Included in
Bioinformatics Commons, Biomedical Informatics Commons, Medical Sciences Commons, Oncology Commons
Comments
Associated Data