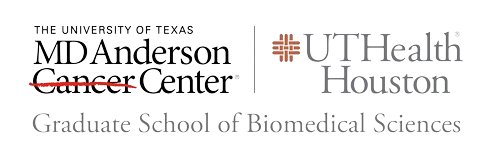
Faculty, Staff and Student Publications
Publication Date
4-1-2023
Journal
IEEE Transactions on Medical Imaging
Abstract
Medical imaging deep learning models are often large and complex, requiring specialized hardware to train and evaluate these models. To address such issues, we propose the PocketNet paradigm to reduce the size of deep learning models by throttling the growth of the number of channels in convolutional neural networks. We demonstrate that, for a range of segmentation and classification tasks, PocketNet architectures produce results comparable to that of conventional neural networks while reducing the number of parameters by multiple orders of magnitude, using up to 90% less GPU memory, and speeding up training times by up to 40%, thereby allowing such models to be trained and deployed in resource-constrained settings.
Keywords
Neural network, segmentation, pattern recognition and classification
DOI
10.1109/TMI.2022.3224873
PMID
36427285
PMCID
PMC10882585
PubMedCentral® Posted Date
February 2024
PubMedCentral® Full Text Version
Author MSS
Published Open-Access
yes
Included in
Bioinformatics Commons, Biomedical Informatics Commons, Medical Sciences Commons, Neurology Commons, Oncology Commons, Radiation Medicine Commons