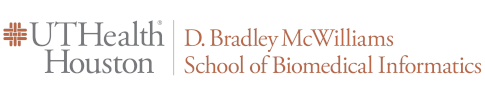
Dissertations & Theses (Open Access)
Graduation Date
Fall 2021
Degree Name
Doctor of Philosophy (PhD)
School Name
The University of Texas School of Biomedical Informatics at Houston
Advisory Committee
Degui Zhi, PhD
Abstract
With the wide adoption of electronic health records (EHRs), researchers, as well as large healthcare organizations, governmental institutions, insurance, and pharmaceutical companies have been interested in leveraging this rich clinical data source to extract clinical evidence and develop predictive algorithms. Large vendors have been able to compile structured EHR data from sites all over the United States, de-identify these data, and make them available to data science researchers in a more usable format. For this dissertation, we leveraged one of the earliest and largest secondary EHR data sources and conducted three studies of increasing scope. In the first study, which was of limited scope, we conducted a retrospective observational study to compare the effect of three drugs on a specific population of approximately 3,000 patients. Using a novel statistical method, we found evidence that the selection of phenylephrine as the primary vasopressor to induce hypertension for the management of nontraumatic subarachnoid hemorrhage is associated with better outcomes as compared to selecting norepinephrine or dopamine. In the second study, we widened our scope, using a cohort of more than 100,000 patients to train generalizable models for the risk prediction of specific clinical events, such as heart failure in diabetes patients or pancreatic cancer. In this study, we found that recurrent neural network-based predictive models trained on expressive terminologies, which preserve a high level of granularity, are associated with better prediction performance as compared with other baseline methods, such as logistic regression. Finally, we widened our scope again, to train Med-BERT, a foundation model, on more than 20 million patients’ diagnosis data. Med-BERT was found to improve the prediction performance of downstream tasks that have a small sample size, which otherwise would limit the ability of the model to learn good representation. In conclusion, we found that we can extract useful information and train helpful deep learning-based predictive models. Given the limitations of secondary EHR data and taking into consideration that the data were originally collected for administrative and not research purposes, however, the findings need clinical validation. Therefore, clinical trials are warranted to further validate any new evidence extracted from such data sources before updating clinical practice guidelines. The implementability of the developed predictive models, which are in an early development phase, also warrants further evaluation.
Recommended Citation
Bekhet, Laila, "Secondary use of Structured Electronic Health Records Data: From Observational Studies to Deep Learning-based Predictive Modeling" (2021). Dissertations & Theses (Open Access). 53.
https://digitalcommons.library.tmc.edu/uthshis_dissertations/53
Keywords
electronic health records, unified medical language system, Med-BERT, predictive modeling, Deep Learning, subarachnoid hemorrhage, phenylephrine, logistic regression, recurrent neural network
Comments
This dissertation has been published in 3 journals:
1. Williams G, Maroufy V, Rasmy L, Brown D, Yu D, Zhu H, Talebi Y, Wang X, Thomas E, Zhu G, Yaseen A, Miao H, Leon Novelo L, Zhi D, DeSantis SM, Zhu H, Yamal JM, Aguilar D, Wu H. Vasopressor treatment and mortality following nontraumatic subarachnoid hemorrhage: a nationwide electronic health record analysis. Neurosurg Focus. 2020 May 1;48(5):E4. doi: 10.3171/2020.2.FOCUS191002. PMID: 32357322.
2. Rasmy L, Tiryaki F, Zhou Y, Xiang Y, Tao C, Xu H, Zhi D. Representation of EHR data for predictive modeling: a comparison between UMLS and other terminologies. J Am Med Inform Assoc. 2020 Oct 1;27(10):1593-1599. doi: 10.1093/jamia/ocaa180. PMID: 32930711; PMCID: PMC7647355.
3. Rasmy L, Xiang Y, Xie Z, Tao C, Zhi D. Med-BERT: pretrained contextualized embeddings on large-scale structured electronic health records for disease prediction. NPJ Digit Med. 2021 May 20;4(1):86. doi: 10.1038/s41746-021-00455-y. PMID: 34017034; PMCID: PMC8137882.