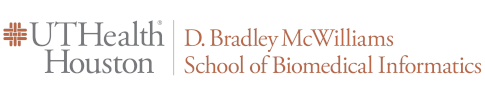
Dissertations & Theses (Open Access)
Graduation Date
Spring 2022
Degree Name
Doctor of Philosophy (PhD)
School Name
The University of Texas School of Biomedical Informatics at Houston
Advisory Committee
Cui Tao, PhD
Abstract
The emergence of deep-learning algorithms provides great potential to enhance the prediction performance of computer-aided supporting diagnosis systems. Recent research efforts indicated that well-trained algorithms could achieve the accuracy level of experienced senior clinicians in the Dermatology field. However, the lack of interpretability and transparency hinders the algorithms’ utility in real-life. Physicians and patients require a certain level of interpretability for them to accept and trust the results. Another limitation of AI algorithms is the lack of consideration of other information related to the disease diagnosis, for example some typical dermoscopic features and diagnostic guidelines. Clinical guidelines for skin disease diagnosis are designed based on dermoscopic features. However, a structured and standard representation of the relevant knowledge in the skin disease domain is lacking.
To address the above challenges, this dissertation builds an ontology capable of formally representing the knowledge of dermoscopic features and develops an explainable deep learning model able to diagnose skin diseases and dermoscopic features. Additionally, large-scale, unlabeled datasets can learn from the trained model and automate the feature generation process. The computer vision aided feature extraction algorithms are combined with the deep learning model to improve the overall classification accuracy and save manual annotation efforts.
Recommended Citation
Zhang, Xinyuan, "Computer-Aided Diagnosis for Melanoma using Ontology and Deep Learning Approaches" (2022). Dissertations & Theses (Open Access). 54.
https://digitalcommons.library.tmc.edu/uthshis_dissertations/54
Keywords
Deep learning, melanoma, image classification, Dermoscopy Elements Visual Ontology, interpretability, dermoscopy, taxonomic relations, diagnostic rules, convolutional neural networks