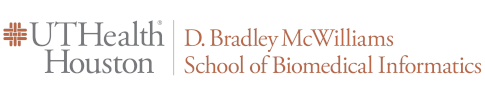
Dissertations & Theses (Open Access)
Graduation Date
Spring 2024
Degree Name
Doctor of Philosophy (PhD)
School Name
McWilliams School of Biomedical Informatics at UTHealth Houston
Advisory Committee
Degui Zhi, PhD
Abstract
It is well recognized that the effectiveness of radiotherapy (RT) requires an intact adaptive immune system, while lymphocytes are highly radiosensitive and are prevalent in the “dose bath” of radiation surrounding the tumor volume. Furthermore, it has been shown that, for nearly all disease sites, radiation-induced lymphopenia (RIL) is associated with poor outcomes. Recently, we observed compelling evidence of significant differences in the lymphocyte-sparing effects of proton therapy (PT) vs. photon (or x-ray) therapy (XRT) attributable to the differences in magnitudes of the dose baths of the two modalities. In addition, previous work has further demonstrated that both patient-specific and dosimetric factors contribute to the risk of severe RIL. However, the implications of these findings for clinical practice remain unknown and require interrogation of RIL as a potential causal mediator of clinical endpoints. Moreover, clinical translation requires predictive risk models and mitigation strategies employing optimization of PT and XRT treatment plans incorporating such models.
To address these problems, this dissertation focuses on (1) severe RIL serves as the mediator of overall survival, while treatment modality contributes significantly towards clinical endpoints; (2) establishing the causal treatment determinants of RIL from dosimetric (including fractionation) characteristics of PT vs. XRT, taking into consideration the heterogeneity of patient-specific clinical and prognostic factors and developing and validating clinically relevant individualized predictive risk models of RIL for esophagus patients with external validation using lung and pancreas cancer datasets; (3) establish optimal individualized lymphopenia-mitigating strategies through the optimization of dose distributions, fractionation regimens and selection of treatment modality; (4) develop lymphocyte depletion model in statistical and deep learning approaches as adaptive RIL risk predictor during treatment.
Our central hypotheses are that (1) a patient’s risk for RIL varies in accordance with quantifiable features of dose distributions, tumor characteristics, and clinical prognostic factors; (2) reducing the dose to circulating immune cells and immune structures at risk preserves not only the quantity but more importantly, the quality of lymphocytes, which maintains cancer immunity and thereby mediates the efficacy of RT; and (3) dosimetric constraints, individualized to the patient’s clinical and tumor characteristics, incorporated into intensity-modulated proton and photon RT (IMPT and IMRT) optimization would mitigate RIL and allow selection of the more appropriate of the proton or photon modalities, yielding evidence-based approaches for personalizing care.
Recommended Citation
Chu, Yan, "Radiation-Induced Lymphopenia: Understanding, Predictive Modelling and Developing Photon and Proton-Based Mitigation Strategies" (2024). Dissertations & Theses (Open Access). 59.
https://digitalcommons.library.tmc.edu/uthshis_dissertations/59
Keywords
Photon and Proton radiation, lymphopenia, deep learning, non-negative Matrix Factorization, composite dosimetric score, Time Series Transformer, lymphocyte depletion forecasting
Comments
This dissertation has been published in the following journal article: Personalized Composite Dosimetric Score-Based Machine Learning Model of Severe Radiation-Induced Lymphopenia among Esophageal Cancer Patients PMID: 38797500.