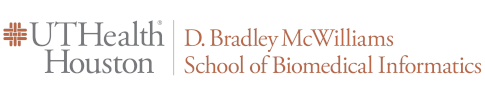
Dissertations & Theses (Open Access)
Graduation Date
Fall 2024
Degree Name
Doctor of Philosophy (PhD)
School Name
McWilliams School of Biomedical Informatics at UTHealth Houston
Advisory Committee
Luca Giancardo, PhD
Abstract
Fundus photography is a radiation-free, non-invasive imaging modality widely employed to assess both ocular and systemic diseases in population-level applications. Traditional methods for extracting cardiovascular biomarkers rely on quantifying vascular features in these images to identify indicators of disease. However, these approaches often depend on manual clinical expertise, which can be costly and time-consuming, making them impractical for large-scale datasets. Deep learning offers a compelling alternative to traditional techniques by enabling the automated processing of extensive datasets with high accuracy. Despite its advantages, deep learning models often function as "black boxes," making it challenging to interpret the features they prioritize or to guide them toward clinically relevant information. To address these challenges, we have focused on adapting deep learning methodologies specifically to emphasize vascular characteristics in retinal images. The development of vasculature-specific models aims to combine the precision of traditional biomarker extraction techniques with the self-learning capabilities of deep learning. This approach enables the detection and characterization of biomarkers associated with diseases such as stroke and diabetes, as well as risk factors like age and sex. In this work, we construct distinct vasculature-specific models to address different tasks within the realm of fundus photography and analysis of disease. Among our contributions, we leveraged optical coherence tomography angiography (OCT-A) data to enhance the vascular visualizations of fundus photos through deep learning. In addition, we further explored the added value of deep learning models directed to the vasculature, by applying them in the detection of disease phenotypes from fundus photography. The results showed some key advantages of vasculature-specific models. We observed that it might be beneficial to train deep learning models using information from the OCT-A images, as it might help them better capture microvascular information from fundus photos. We also observed that extracting and encoding features specific to the retinal vessels from the fundus photographs, not only directs deep learning models towards relevant vascular information but permits creating general-purpose representations that require less data than out-of-the-box approaches and can be easily tested across different outcomes. Overall, we deliver a variety of techniques for the analysis of vascular information from fundus photographs. We would like these techniques to provide a foundation for integrating deep learning into vascular biomarker discovery and improve the understanding of disease mechanisms through retinal imaging.
Recommended Citation
Coronado, Ivan, "Cardiovascular Biomarkers using Deep Learning and Retinal Imaging for the Assessment of Systemic Disease" (2024). Dissertations & Theses (Open Access). 67.
https://digitalcommons.library.tmc.edu/uthshis_dissertations/67
Keywords
Deep learning, cardiovascular and retinal biomarkers, Fundus photos, 6. Optical Coherence Tomography Angiography (OCT-A), Stroke Detection, Diabetes Detection