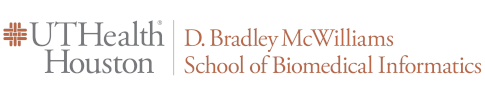
Faculty, Staff and Student Publications
Publication Date
12-12-2022
Journal
JMIR Dermatology
Abstract
BACKGROUND: Automatic skin lesion recognition has shown to be effective in increasing access to reliable dermatology evaluation; however, most existing algorithms rely solely on images. Many diagnostic rules, including the 3-point checklist, are not considered by artificial intelligence algorithms, which comprise human knowledge and reflect the diagnosis process of human experts.
OBJECTIVE: In this paper, we aimed to develop a semisupervised model that can not only integrate the dermoscopic features and scoring rule from the 3-point checklist but also automate the feature-annotation process.
METHODS: We first trained the semisupervised model on a small, annotated data set with disease and dermoscopic feature labels and tried to improve the classification accuracy by integrating the 3-point checklist using ranking loss function. We then used a large, unlabeled data set with only disease label to learn from the trained algorithm to automatically classify skin lesions and features.
RESULTS: After adding the 3-point checklist to our model, its performance for melanoma classification improved from a mean of 0.8867 (SD 0.0191) to 0.8943 (SD 0.0115) under 5-fold cross-validation. The trained semisupervised model can automatically detect 3 dermoscopic features from the 3-point checklist, with best performances of 0.80 (area under the curve [AUC] 0.8380), 0.89 (AUC 0.9036), and 0.76 (AUC 0.8444), in some cases outperforming human annotators.
CONCLUSIONS: Our proposed semisupervised learning framework can help with the automatic diagnosis of skin disease based on its ability to detect dermoscopic features and automate the label-annotation process. The framework can also help combine semantic knowledge with a computer algorithm to arrive at a more accurate and more interpretable diagnostic result, which can be applied to broader use cases.
Keywords
deep learning, dermoscopic images, semisupervised learning, 3-point checklist, skin lesion, dermatology, algorithm, melanoma classification, melanoma, automatic diagnosis, skin disease
Included in
Biomedical Informatics Commons, Dermatology Commons, Skin and Connective Tissue Diseases Commons
Comments
PMID: 37632881