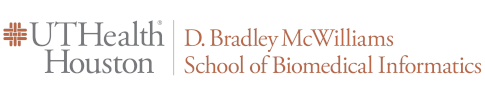
Faculty, Staff and Student Publications
Publication Date
6-1-2022
Journal
Journal of Biomedical Informatics
Abstract
OBJECTIVE: The Coronavirus Disease 2019 (COVID-19) pandemic has overwhelmed the capacity of healthcare resources and posed a challenge for worldwide hospitals. The ability to distinguish potentially deteriorating patients from the rest helps facilitate reasonable allocation of medical resources, such as ventilators, hospital beds, and human resources. The real-time accurate prediction of a patient's risk scores could also help physicians to provide earlier respiratory support for the patient and reduce the risk of mortality.
METHODS: We propose a robust real-time prediction model for the in-hospital COVID-19 patients' probability of requiring mechanical ventilation (MV). The end-to-end neural network model incorporates the Multi-task Gaussian Process to handle the irregular sampling rate in observational data together with a self-attention neural network for the prediction task.
RESULTS: We evaluate our model on a large database with 9,532 nationwide in-hospital patients with COVID-19. The model demonstrates significant robustness and consistency improvements compared to conventional machine learning models. The proposed prediction model also shows performance improvements in terms of area under the receiver operating characteristic curve (AUROC) and area under the precision-recall curve (AUPRC) compared to various deep learning models, especially at early times after a patient's hospital admission.
CONCLUSION: The availability of large and real-time clinical data calls for new methods to make the best use of them for real-time patient risk prediction. It is not ideal for simplifying the data for traditional methods or for making unrealistic assumptions that deviate from observation's true dynamics. We demonstrate a pilot effort to harmonize cross-sectional and longitudinal information for mechanical ventilation needing prediction.
Keywords
Attention, COVID-19, Cross-Sectional Studies, Humans, Neural Networks, Computer, Retrospective Studies, Ventilators, Mechanical
DOI
10.1016/j.jbi.2022.104079
PMID
35489596
PMCID
PMC9044651
PubMedCentral® Posted Date
April 2022
PubMedCentral® Full Text Version
Post-Print
Comments
Supplementary Materials