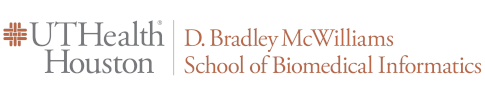
Faculty, Staff and Student Publications
Publication Date
9-11-2023
Journal
Genome Biology
Abstract
Growing regulatory requirements set barriers around genetic data sharing and collaborations. Moreover, existing privacy-aware paradigms are challenging to deploy in collaborative settings. We present COLLAGENE, a tool base for building secure collaborative genomic data analysis methods. COLLAGENE protects data using shared-key homomorphic encryption and combines encryption with multiparty strategies for efficient privacy-aware collaborative method development. COLLAGENE provides ready-to-run tools for encryption/decryption, matrix processing, and network transfers, which can be immediately integrated into existing pipelines. We demonstrate the usage of COLLAGENE by building a practical federated GWAS protocol for binary phenotypes and a secure meta-analysis protocol. COLLAGENE is available at https://zenodo.org/record/8125935.
Keywords
Collaborative analysis, Federated model training, Genomic data privacy, Data security
DOI
10.1186/s13059-023-03039-z
PMID
37697426
PMCID
PMC10496350
PubMedCentral® Posted Date
September 2023
PubMedCentral® Full Text Version
Post-Print
Included in
Biomedical Informatics Commons, Genetic Phenomena Commons, Genetic Processes Commons, Medical Genetics Commons
Comments
Supplementary Materials