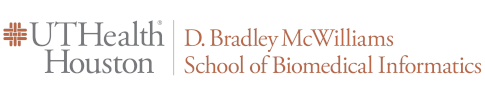
Faculty, Staff and Student Publications
Publication Date
1-1-2023
Journal
AMIA Summits on Translational Science Proceedings
Abstract
Self-controlled case series (SCCS) is a statistical method in epidemiological study design that uses individuals as their own controls, with comparisons made within the same individuals at different time points of observation. SCCS has been applied in settings where it is difficult to identify comparison or control groups. To provide computational support for SCCS, we introduce a query engine called Self-Controlled Case Query (SCCQ) and use it to extract cohorts of self-controlled case series from a large-scale COVID-19 Electronic Health Records (EHR) dataset. Visual summary of the queried population through the R-Shiny visualization framework offers SCCQ's query result dashboard to the researcher. SCCQ allows the export of query-generated raw data files with a portable format that researchers can extend to create more intricate and robust visualization capabilities without needing a high-level of technical or statistical background. Our validation and evaluation experiments uncovered COVID-19 outcomes to be consistent with existing research findings. With SCCQ, cohort exploration, data extraction, and information visualization can be provided for structured EHR data to lower the barrier for clinical and epidemiological research.
PMID
37350916
PMCID
PMC10283100
PubMedCentral® Posted Date
June 2023
PubMedCentral® Full Text Version
Post-Print
Comments
PMID: 37350916