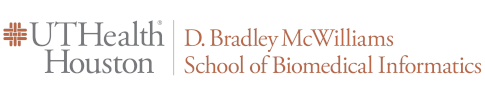
Faculty, Staff and Student Publications
Publication Date
6-1-2023
Journal
Bioinformatics
Abstract
MOTIVATION: Single-cell RNA sequencing enables researchers to study cellular heterogeneity at single-cell level. To this end, identifying cell types of cells with clustering techniques becomes an important task for downstream analysis. However, challenges of scRNA-seq data such as pervasive dropout phenomena hinder obtaining robust clustering outputs. Although existing studies try to alleviate these problems, they fall short of fully leveraging the relationship information and mainly rely on reconstruction-based losses that highly depend on the data quality, which is sometimes noisy.
RESULTS: This work proposes a graph-based prototypical contrastive learning method, named scGPCL. Specifically, scGPCL encodes the cell representations using Graph Neural Networks on cell-gene graph that captures the relational information inherent in scRNA-seq data and introduces prototypical contrastive learning to learn cell representations by pushing apart semantically dissimilar pairs and pulling together similar ones. Through extensive experiments on both simulated and real scRNA-seq data, we demonstrate the effectiveness and efficiency of scGPCL.
AVAILABILITY AND IMPLEMENTATION: Code is available at https://github.com/Junseok0207/scGPCL.
Keywords
Gene Expression Profiling, Software, Sequence Analysis, RNA, Single-Cell Gene Expression Analysis, Single-Cell Analysis, Cluster Analysis
PMID
37233193
PMCID
PMC10246584
PubMedCentral® Posted Date
5-26-2023
PubMedCentral® Full Text Version
Post-print
Published Open-Access
yes
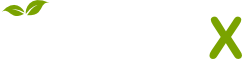
- Citations
- Citation Indexes: 5
- Usage
- Downloads: 22
- Abstract Views: 5
- Captures
- Readers: 13
- Mentions
- News Mentions: 2
Included in
Bioinformatics Commons, Biomedical Informatics Commons, Genetic Processes Commons, Health Information Technology Commons, Medical Genetics Commons, Medical Specialties Commons