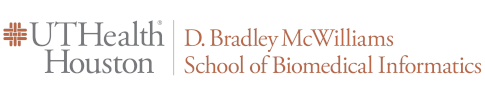
Faculty, Staff and Student Publications
Publication Date
7-1-2023
Journal
Journal of Biomedical Informatics
Abstract
The emphasis on fairness in predictive healthcare modeling has increased in popularity as an approach for overcoming biases in automated decision-making systems. The aim is to guarantee that sensitive characteristics like gender, race, and ethnicity do not influence prediction outputs. Numerous algorithmic strategies have been proposed to reduce bias in prediction results, mitigate prejudice toward minority groups and promote prediction fairness. The goal of these strategies is to ensure that model prediction performance does not exhibit significant disparity among sensitive groups. In this study, we propose a novel fairness-achieving scheme based on multitask learning, which fundamentally differs from conventional fairness-achieving techniques, including altering data distributions and constraint optimization through regularizing fairness metrics or tampering with prediction outcomes. By dividing predictions on different sub-populations into separate tasks, we view the fairness problem as a task-balancing problem. To ensure fairness during the model-training process, we suggest a novel dynamic re-weighting approach. Fairness is achieved by dynamically modifying the gradients of various prediction tasks during neural network back-propagation, and this novel technique applies to a wide range of fairness criteria. We conduct tests on a real-world use case to predict sepsis patients' mortality risk. Our approach satisfies that it can reduce the disparity between subgroups by 98% while only losing less than 4% of prediction accuracy.
Keywords
Humans, Learning, Benchmarking, Minority Groups, Neural Networks, Computer, Sepsis
DOI
10.1016/j.jbi.2023.104399
PMID
37211197
PMCID
PMC10665114
PubMedCentral® Posted Date
11-22-2023
PubMedCentral® Full Text Version
Author MSS
Published Open-Access
yes