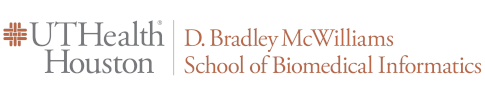
Journal Articles
Publication Date
4-1-2023
Journal
JCO Clinical Cancer Informatics
Abstract
PURPOSE: Early detection of brain metastases (BMs) is critical for prompt treatment and optimal control of the disease. In this study, we seek to predict the risk of developing BM among patients diagnosed with lung cancer on the basis of electronic health record (EHR) data and to understand what factors are important for the model to predict BM development through explainable artificial intelligence approaches accurately.
MATERIALS AND METHODS: We trained a recurrent neural network model, REverse Time AttentIoN (RETAIN), to predict the risk of developing BM using structured EHR data. To interpret the model's decision process, we analyzed the attention weights in the RETAIN model and the SHAP values from a feature attribution method, Kernel SHAP, to identify the factors contributing to BM prediction.
RESULTS: We developed a high-quality cohort with 4,466 patients with BM from the Cerner Health Fact database, which contains over 70 million patients from more than 600 hospitals. RETAIN uses this data set to achieve the best area under the receiver operating characteristic curve at 0.825, a significant improvement over the baseline model. We also extended a feature attribution method, Kernel SHAP, to structured EHR data for model interpretation. Both RETAIN and Kernel SHAP can identify important features related to BM prediction.
CONCLUSION: To the best of our knowledge, this is the first study to predict BM using structured EHR data. We achieved decent prediction performance for BM prediction and identified factors highly relevant to BM development. The sensitivity analysis demonstrated that both RETAIN and Kernel SHAP could discriminate unrelated features and put more weight on the features important to BM. Our study explored the potential of applying explainable artificial intelligence for future clinical applications.
Keywords
Humans, Artificial Intelligence, Electronic Health Records, Early Detection of Cancer, Lung Neoplasms, Brain Neoplasms
Included in
Biomedical Informatics Commons, Health Information Technology Commons, Medical Sciences Commons
Comments
PMID: 37018650