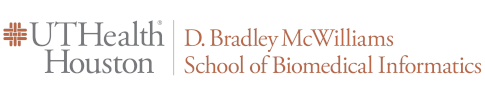
Faculty, Staff and Student Publications
Publication Date
1-20-2023
Journal
iScience
Abstract
Developing drugs for treating Alzheimer's disease has been extremely challenging and costly due to limited knowledge of underlying mechanisms and therapeutic targets. To address the challenge in AD drug development, we developed a multi-task deep learning pipeline that learns biological interactions and AD risk genes, then utilizes multi-level evidence on drug efficacy to identify repurposable drug candidates. Using the embedding derived from the model, we ranked drug candidates based on evidence from post-treatment transcriptomic patterns, efficacy in preclinical models, population-based treatment effects, and clinical trials. We mechanistically validated the top-ranked candidates in neuronal cells, identifying drug combinations with efficacy in reducing oxidative stress and safety in maintaining neuronal viability and morphology. Our neuronal response experiments confirmed several biologically efficacious drug combinations. This pipeline showed that harmonizing heterogeneous and complementary data/knowledge, including human interactome, transcriptome patterns, experimental efficacy, and real-world patient data shed light on the drug development of complex diseases.
Keywords
Drugs, Neuroscience, Artificial intelligence
DOI
10.1016/j.isci.2022.105678
PMID
36594024
PMCID
PMC9804117
PubMedCentral® Posted Date
11-26-2022
PubMedCentral® Full Text Version
Post-print
Published Open-Access
yes
Graphical Abstract
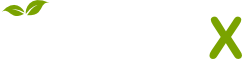
- Citations
- Citation Indexes: 10
- Usage
- Downloads: 1
- Abstract Views: 1
- Captures
- Readers: 46
- Mentions
- News Mentions: 1
Included in
Artificial Intelligence and Robotics Commons, Bioinformatics Commons, Biomedical Informatics Commons, Data Science Commons, Neurosciences Commons